Depression classification model on Twitter using BERT
Abstract
Today there are many signs of depression, as well as many suicide attempts caused by this emotional disorder, and this is reflected mostly on social networks, mainly on Twitter. For this reason, it is important for specialists and organizations seeking to safeguard people's lives to use software tools to address this problem. For this, in this work a web tool called "UBDevs-Depression-Classifier" is proposed, that allows you to automatically obtain and classify tweets for a specific topic. A greater emphasis was placed on tweets related to COVID-19in the years 2020-2021 the world experienced a pandemic that increased cases of depression in many places. This research proposal focuses on the use of a model based on NLP (Natural Language Processing) for the classification of Tweets in order to find those that incite depression or imply that users are in a bad mood, all this in order to maintain the mental and physical health of the users of this platform. There are several models that are used as a basis for NLP projects, however, at present BERT has proven to be one of the most efficient, so we selected it for the development of our proposal. To evaluate the efficiency of the project we applied the F1 metric obtaining a value of 0.8806, a quite acceptable result with respect to a textual classification.
Downloads
References
Chen, F., Zheng, D., Liu, J., Gong, Y., Guan, Z., & Lou, D. (2020). Depression and anxiety among adolescents during COVID-19: A cross-sectional study. Brain, behavior, and immunity, 88, 36.
Islam, M. A., Barna, S. D., Raihan, H., Khan, M. N. A., & Hossain, M. T. (2020). Depression and anxiety among university students during the COVID-19 pandemic in Bangladesh: A web-based cross-sectional survey. PloS one, 15(8), e0238162.
Lee, S. A., Jobe, M. C., Mathis, A. A., & Gibbons, J. A. (2020). Incremental validity of coronaphobia: Coronavirus anxiety explains depression, generalized anxiety, and death anxiety. Journal of anxiety disorders, 74, 102268.
Santini, Z. I., Jose, P. E., Cornwell, E. Y., Koyanagi, A., Nielsen, L., Hinrichsen, C., ... & Koushede, V. (2020). Social disconnectedness, perceived isolation, and symptoms of depression and anxiety among older Americans (NSHAP): a longitudinal mediation analysis. The Lancet Public Health, 5(1), e62-e70.
Bhuiyan, A. I., Sakib, N., Pakpour, A. H., Griffiths, M. D., & Mamun, M. A. (2020). COVID-19-related suicides in Bangladesh due to lockdown and economic factors: case study evidence from media reports. International Journal of Mental Health and Addiction, 1-6.
Reddy MS. Depression - the global crisis. Indian J Psychol Med 2012;34:201-3.
World Health Organization. (2014). Preventing suicide: A global imperative. World Health Organization.
Charoensukmongkol, P. (2018). The impact of social media on social comparison and envy in teenagers: The moderating role of the parent comparing children and in-group competition among friends. Journal of Child and Family Studies, 27(1), 69-79.
Anger, I., & Kittl, C. (2011, September). Measuring influence on Twitter. In Proceedings of the 11th international conference on knowledge management and knowledge technologies (pp. 1-4).
Sobrino Sande, J. C. (2018) Análisis de sentimientos en Twitter.
Kauffmann, E., Peral, J., Gil, D., Ferrández, A., Sellers, R., & Mora, H. (2020). A framework for big data analytics in commercial social networks: A case study on sentiment analysis and fake review detection for marketing decision-making. Industrial Marketing Management, 90, 523-537.
Back, B. H., & Ha, I. K. (2019). Comparison of sentiment analysis from large Twitter datasets by Naïve Bayes and natural language processing methods. Journal of information and communication convergence engineering, 17(4), 239-245.
Leis, A., Ronzano, F., Mayer, M. A., Furlong, L. I., & Sanz, F. (2019). Detecting signs of depression in tweets in Spanish: behavioral and linguistic analysis. Journal of medical Internet research, 21(6), e14199.
Singh, M., Jakhar, A. K., & Pandey, S. (2021). Sentiment analysis on the impact of coronavirus in social life using the BERT model. Social Network Analysis and Mining, 11(1), 1-11.
Chiorrini, A., Diamantini, C., Mircoli, A., & Potena, D. (2021). Emotion and sentiment analysis of tweets using BERT. In EDBT/ICDT Workshops.
Pota, M., Ventura, M., Fujita, H., & Esposito, M. (2021). Multilingual evaluation of pre-processing for BERT-based sentiment analysis of tweets. Expert Systems with Applications, 181, 115119.
Qadeer, S., & Wu, D. (2004). KISS: keep it simple and sequential. ACM sigplan notices, 39(6), 14-24.
Rotge, J. F. (2000, September). SGDL-Scheme: a high level algorithmic language for projective solid modeling programming. In Proceedings of the Scheme and Functional Programming 2000 Workshop (Montreal, Canada (pp. 31-34).
Devlin, J., Chang, M. W., Lee, K., & Toutanova, K. (2018). Bert: Pre-training of deep bidirectional transformers for language understanding. arXiv preprint arXiv:1810.04805.
Tan, P., Steinbach, M., and Kumar, V. (2013).Introduction to Data Mining: Pearson NewInternational Edition. Pearson Education Limited.
Virahonda, S. (2021). Depressive and Anxious Tweets. Disponible en: https://www.kaggle.com/datasets/sergiovirahonda/depression-anxiety-tweets
Kazanova, M. (2018). Sentiment140 dataset with 1.6 million tweets. Disponible en: https://www.kaggle.com/datasets/kazanova/sentiment140
Hatzivassiloglou, V., & McKeown, K. R. (1997, July). Predicting the semantic orientation of adjectives. In Proceedings of the 35th annual meeting of the association for computational linguistics and eighth conference of the european chapter of the association for computational linguistics (pp. 174-181). Association for Computational Linguistics
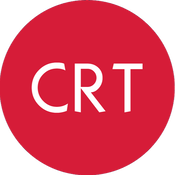
- Conceptualization
- Data curation
- Formal Analysis
- Investigation
- Methodology
- Software
- Validation
- Visualization
- Writing - original draft
- Writing - review & editing
- Conceptualization
- Data curation
- Investigation
- Methodology
- Software
- Validation
- Visualization
- Writing - original draft
- Conceptualization
- Data curation
- Formal Analysis
- Investigation
- Methodology
- Software
- Validation
- Visualization
- Writing - original draft
- Writing - review & editing
Copyright (c) 2023 Innovation and Software

This work is licensed under a Creative Commons Attribution 4.0 International License.
The authors exclusively grant the right to publish their article to the Innovation and Software Journal, which may formally edit or modify the approved text to comply with their own editorial standards and with universal grammatical standards, prior to publication; Likewise, our journal may translate the approved manuscripts into as many languages as it deems necessary and disseminates them in several countries, always giving public recognition to the author or authors of the research.