Recognition and Classification of Hate Messages
Abstract
The massive use of social networks and the anonymity that this provides has made possible not only the immediate communication between users, but also the spread of hate speech against certain groups of our society in the form of offensive messages to them, this has led to a serious social problem, which remains a topic of current research along with NLP. The purpose of the present work is to make a comparison of our "HateCheck" recognition model against the author's results, using the same database as them. To do so, we will make use of the main metrics such as: precision, recall and F1.
Downloads
References
G. Eason, B. Noble, and I. N. Sneddon, “On certain integrals of Lipschitz-Hankel type involving products of Bessel functions,” Phil. Trans. Roy. Soc. London, vol. A247, pp. 529–551, April 1955.
VAN AKEN, Betty, et al., “Challenges for toxic comment classification: An in-depth error analysis“, 2018.
BANKO, Michele; MACKEEN, Brendon, “RAY, Laurie. A unified taxonomy of harmful content. En Proceedings of the fourth workshop on online abuse and harms“, 2020, p. 125-137.
PLAZA-DEL-ARCO, Flor Miriam, et al. “Comparing pretrained language models for Spanish hate speech detection. Expert Systems with Applications“, 2021, vol. 166, p. 114120.
Thomas Davidson, Dana Warmsley, Michael Macy and Ingmar Weber “Automated hate speech detection and the problem of offensive languaje“. In Proceedings of the 11th International AAAI Conference on Web and Social Media, 2017, p. 512-515
Alexis Palmer, Christine Carr, Melissa Robinson, Jordan Sanders. “COLD: Annotation scheme and evaluation data set for complex offensive language in English“, Journal for Language Technology and Computational Linguistics, p. 1-28.
Alexis Palmer, Christine Carr, Melissa Robinson, Jordan Sanders. “COLD: Annotation scheme and evaluation data set for complex offensive language in English“, Journal for Language Technology and Computational Linguistics, p. 1-28.
B. Lohitha, M. V and J. J. Amarnath, "A Comparison of Different Models for the Detection of Hate Speech," 2022 1st International Conference on Computational Science and Technology (ICCST), CHENNAI, India, 2022, pp. 492-496, doi: 10.1109/ICCST55948.2022.10040400.
RÖTTGER, Paul, et al. HateCheck: Functional tests for hate speech detection models. arXiv preprint arXiv:2012.15606, 2020.
BALKIR, Esma, et al. Necessity and sufficiency for explaining text classifiers: A case study in hate speech detection. arXiv preprint arXiv:2205.03302, 2022.
OTTER, Daniel W.; MEDINA, Julian R.; KALITA, Jugal K. A survey of the usages of deep learning for natural language processing. IEEE transactions on neural networks and learning systems, 2020, vol. 32, no 2, p. 604-624.
WU, Stephen, et al. Deep learning in clinical natural language processing: a methodical review. Journal of the American Medical Informatics Association, 2020, vol. 27, no 3, p. 457-470.
SHISHAH, Wesam; FAJRI, Ricky Maulana. Large Comparative Study of Recent Computational Approach in Automatic Hate Speech Detection. TEM Journal, 2022, vol. 11, no 1, p. 82.
PÉREZ, Juan Manuel, et al. Assessing the impact of contextual information in hate speech detection. IEEE Access, 2023, vol. 11, p. 30575-30590.
RÖTTGER, Paul, et al. MULTILINGUAL HATECHECK: Functional Tests for Multilingual Hate Speech Detection Models. arXiv preprint arXiv:2206.09917, 2022.
ABRO, Sindhu, et al. Automatic hate speech detection using machine learning: A comparative study. International Journal of Advanced Computer Science and Applications, 2020, vol. 11, no 8.
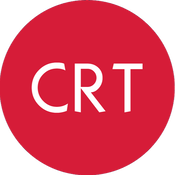
- Conceptualization
- Data curation
- Formal Analysis
- Investigation
- Methodology
- Software
- Validation
- Visualization
- Writing - original draft
- Writing - review & editing
- Conceptualization
- Data curation
- Formal Analysis
- Investigation
- Methodology
- Software
- Validation
- Visualization
- Writing - original draft
- Writing - review & editing
- Conceptualization
- Data curation
- Formal Analysis
- Investigation
- Methodology
- Software
- Validation
- Visualization
- Writing - original draft
- Writing - review & editing
Copyright (c) 2023 Innovation and Software

This work is licensed under a Creative Commons Attribution 4.0 International License.
The authors exclusively grant the right to publish their article to the Innovation and Software Journal, which may formally edit or modify the approved text to comply with their own editorial standards and with universal grammatical standards, prior to publication; Likewise, our journal may translate the approved manuscripts into as many languages as it deems necessary and disseminates them in several countries, always giving public recognition to the author or authors of the research.