Predictive model for the early detection of students with high risk of academic dropout
Abstract
The results of 4 predictive models, logistic regression, decision trees, KNN and a neural network are compared to predict the academic dropout of students at the National Intercultural University of the Amazon, applied to a dataset extracted from the system's database. of academic management of the university, which contains socioeconomic and academic performance data which were processed and formatted using onehotencoding techniques in order to apply the predictive models already mentioned. For data processing and formatting, Transac Sql queries were used and the application of predictive models was done through Knime Software and using Python through Google Colab. The results obtained by applying 4 predictive models are very good since they all exceeded 80% of Accuracy, which guarantees that they can be put into production for the benefit of the university and thus can make better decisions when addressing academic dropout. . It is concluded that applying a predictive model in universities for the early detection of students with high risk of academic dropout is viable and very beneficial so that universities, through their academic managers, can apply more focused strategies to reduce their academic dropout rates.
Downloads
References
T. Viale, «Enfoque UPC,» 10 enero 2020. [En línea]. Available: https://enfoque.upc.edu.pe/mas-temas/educacion/desercion-estudiantil-universitaria-accionamos-o-reaccionamos/.
uPlanner, «uPlanner,» 27 Marzo 2017. [En línea]. Available: https://uplanner.com/es/blog/8-causas-de-desercion-estudiantil-en-la-educacion-superior/.
Gabriel, Jaime, Páramo, Arturo y Correa, Deserción Estudiantil Universitaria. Conceptualización, Revista Universidad Eafit, 1999.
H. Chitarroni, La regresión logística, Buenos Aires: Instituto de Investigación en Ciencias Sociales, 2002.
C. N. Bouza y A. Santiago, MODELACIÓN MATEMÁTICA DE FENÓMENOS DEL MEDIO AMBIENTE Y LA SALUD, Mexico: Universidad Autónoma de Guerrero, 2012.
aprendemachinelearning, «Clasificar con K-Nearest-Neighbor ejemplo en Python,» July 2018. [En línea]. Available: https://www.aprendemachinelearning.com/clasificar-con-k-nearest-neighbor-ejemplo-en-python/.
R. F. López y J. M. F. Fernández, Las Redes Neuronales Artificiales - Fundamentos teoricos y aplicaciones prácticas., España: lorena bello, 2008.
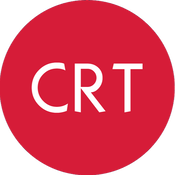
Copyright (c) 2021 Innovation and Software

This work is licensed under a Creative Commons Attribution 4.0 International License.
The authors exclusively grant the right to publish their article to the Innovation and Software Journal, which may formally edit or modify the approved text to comply with their own editorial standards and with universal grammatical standards, prior to publication; Likewise, our journal may translate the approved manuscripts into as many languages as it deems necessary and disseminates them in several countries, always giving public recognition to the author or authors of the research.