Application of decision trees for the identification of adaptability of students in online education
Abstract
Due to the global pandemic by Covid-19, online education was established in student learning. However, the effectiveness of this modality, as well as the adaptability of the students, is something that may depend on some factors. In this sense, this research article presents a description of the use of decision trees to determine the adaptability of students in online education, using a dataset of 1205 records with data such as the type of connection and internet, device, condition. financial, among other important data. Likewise, tools such as Google Colab, Python and popular libraries were used in similar works of Artificial Intelligence and Machine Learning.
Downloads
References
R. P. S. Elizabeth, «PERCEPCIONES DE LA EDUCACIÓN VIRTUAL EN CONFINAMIENTO,» 19 Agosto 2021. [En línea]. Available: http://201.159.222.95/bitstream/123456789/2251/1/RODRIGUEZ%20PONCE%20SUSANA%20ELIZABETH.pdf. [Último acceso: Junio 2022].
M. H. Suzan, «Students Adaptability Level in Online Education,» Abril 2022. [En línea]. Available: https://www.kaggle.com/datasets/mdmahmudulhasansuzan/students-adaptability-level-in-online-education. [Último acceso: Junio 2022].
M. Minsky, «The age of Intelligent Machines: Thoughts About Artificial Intelligence,» KurzweilAl.net., 1990.
Ocaña-Fernández, Yolvi, Luis Alex Valenzuela-Fernández, y Luzmila Lourdes Garro-Aburto. "Artificial Intelligence and Its Implications in Higher Education." Propósitos Y Representaciones 7.2 (2019).
B. Díaz-Landa, R. Meleán-Romero y W. Marín-Rodriguez, "Rendimiento académico de estudiantes en Educación Superior: predicciones de factores influyentes a partir de árboles de decisión", Telos Revista de Estudios Interdisciplinarios en Ciencias Sociales, vol. 23, n.º 3, pp. 616–639, septiembre de 2021. Accedido el 19 de agosto de 2022. [En línea]. Disponible: https://doi.org/10.36390/telos233.08
C. H. Menacho Chiok, "Predicción del rendimiento académico aplicando técnicas de minería de datos", Anales Científicos, vol. 78, n.º 1, p. 26, junio de 2017. Accedido el 19 de agosto de 2022. [En línea]. Disponible: https://doi.org/10.21704/ac.v78i1.811
P. E. Ramírez, «Predicción de la Deserción Académica en una Universidad Pública Chilena a través de la Clasificación basada en Árboles de Decisión con Parámetros Optimizados,» 26 Enero 2018. [En línea]. [Último acceso: Junio 2022].
M. Hasan Suzan, N. A. Samrin, A. A. Biswas y A. Pramanik, "Students' Adaptability Level Prediction in Online Education using Machine Learning Approaches", en 2021 12th International Conference on Computing Communication and Networking Technologies (ICCCNT), Kharagpur, India, 6–8 de julio de 2021. IEEE, 2021. Accedido el 20 de agosto de 2022. [En línea]. Disponible: https://doi.org/10.1109/icccnt51525.2021.9579741
S. T. "Entropy: How Decision Trees Make Decisions". Medium. https://towardsdatascience.com/entropy-how-decision-trees-make-decisions-2946b9c18c8 (accedido el 14 de agosto de 2022).
C. Ayuya. "Entropy and Information Gain to Build Decision Trees in Machine Learning". Engineering Education (EngEd) Program | Section. https://www.section.io/engineering-education/entropy-information-gain-machine-learning/ (accedido el 14 de agosto de 2022).
P. Gupta. "Decision Trees in Machine Learning". Medium. https://towardsdatascience.com/decision-trees-in-machine-learning-641b9c4e8052 (accedido el 14 de agosto de 2022).
Shanna S. Jaggars, «Adaptability to Online Learning: Differences Across Types of Students and Academic Subject Areas» February 2013 [En LInea] https://academiccommons.columbia.edu/doi/10.7916/D82N59NB
M. L Garg , ”Predictive Analytics: A Review of Trends and Techniques” July 2018 [En Linea] https://www.researchgate.net/profile/Vaibhav-Kumar-16/publication/326435728_Predictive_Analytics_A_Review_of_Trends_and_Techniques/links/5c484f6692851c22a38a6027/Predictive-Analytics-A-Review-of-Trends-and-Techniques.pdf
Dazhong W. “Cloud-Based Machine Learning for Predictive Analytics: Tool Wear Prediction in Milling” 2016 [En Linea] https://www.researchgate.net/profile/Soundar-Kumara/publication/313456166_Cloud-Based_Machine_Learning_for_Predictive_Analytics_Tool_Wear_Prediction_in_Milling/links/605fbecc92851cd8ce6fbc07/Cloud-Based-Machine-Learning-for-Predictive-Analytics-Tool-Wear-Prediction-in-Milling.pdf
Tan, C., Lin, J. A new QoE-based prediction model for evaluating virtual education systems with COVID-19 side effects using data mining. Soft Comput (2021). https://doi.org/10.1007/s00500-021-05932-w
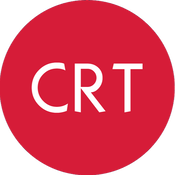
- Conceptualization
- Data curation
- Formal Analysis
- Investigation
- Methodology
- Software
- Validation
- Visualization
- Writing - original draft
- Writing - review & editing
- Conceptualization
- Data curation
- Formal Analysis
- Investigation
- Methodology
- Software
- Validation
- Visualization
- Writing - original draft
- Writing - review & editing
- Conceptualization
- Data curation
- Formal Analysis
- Investigation
- Methodology
- Software
- Validation
- Visualization
- Writing - original draft
- Writing - review & editing
- Conceptualization
- Data curation
- Formal Analysis
- Investigation
- Methodology
- Software
- Validation
- Visualization
- Writing - original draft
- Writing - review & editing
Copyright (c) 2023 Innovation and Software

This work is licensed under a Creative Commons Attribution 4.0 International License.
The authors exclusively grant the right to publish their article to the Innovation and Software Journal, which may formally edit or modify the approved text to comply with their own editorial standards and with universal grammatical standards, prior to publication; Likewise, our journal may translate the approved manuscripts into as many languages as it deems necessary and disseminates them in several countries, always giving public recognition to the author or authors of the research.