La IA Generativa en el Desarrollo de Software: Impacto en Diversas Industrias
Resumen
El desarrollo de software experimentó una transformación significativa mediante la integración de tecnologías de inteligencia artificial (IA) generativa. La investigación se propuso evaluar la influencia de estas tecnologías en diferentes industrias y sus implicaciones en los procesos de desarrollo mediante un análisis sistemático de su impacto e implementación en diversos sectores industriales. Se implementó la metodología PRISMA para el análisis de publicaciones entre 2022 y 2024 en las bases de datos Scopus y SciELO, empleando criterios específicos de inclusión y términos clave relacionados con aplicaciones de IA. El estudio reveló una adopción significativa en las industrias farmacéutica, alimentaria, salud dental y maxilofacial, marítima, minería, telecomunicaciones, salud mental y educación médica, registrándose un incremento sustancial en la producción científica, que evolucionó de 40 documentos en 2022 a 54 en 2024. El análisis geográfico mostró un liderazgo de China en publicaciones Scopus, mientras que Brasil destacó su investigación en SciELO. Los hallazgos demostraron que estas tecnologías optimizaron la eficiencia en la generación y depuración de código, democratizando el desarrollo de software y facilitando la creación de soluciones efectivas sin requerir experiencia técnica avanzada. Se concluyó que, no obstante, los desafíos en precisión e implicaciones éticas, las herramientas basadas en IA generativa se consolidaron como elementos fundamentales para la competitividad organizacional en el entorno tecnológico contemporáneo.
Descargas
Citas
K. Costello, “Gartner Survey of More Than 3,000 CIOs Reveals That Enterprises Are Entering the Third Era of IT,” Gartner.com. Accessed: Dec. 06, 2024. [Online]. Available: https://www.gartner.com/en/newsroom/press-releases/2018-10-16-gartner-survey-of-more-than-3000-cios-reveals-that-enterprises-are-entering-the-third-era-of-it
Bergur Thormundsson, “Global AI software market size 2018-2025.” Mar. 17, 2022. Accessed: Dec. 06, 2024. [PDF]. Available: https://www.statista.com/statistics/607716/worldwide-artificial-intelligence-market-revenues/
B. Yetiştiren, I. Özsoy, M. Ayerdem, and E. Tüzün, “Evaluating the Code Quality of AI-Assisted Code Generation Tools: An Empirical Study on GitHub Copilot, Amazon CodeWhisperer, and ChatGPT,” Oct. 22, 2023, arXiv: arXiv:2304.10778. doi: 10.48550/arXiv.2304.10778.
Aarti, “Generative Ai in Software Development : an Overview and Evaluation of Modern Coding Tools,” Int. J. Multidiscip. Res., vol. 6, no. 3, p. 23271, Jun. 2024, doi: 10.36948/ijfmr.2024.v06i03.23271.
M. A. Florez Muñoz, J. C. Jaramillo De La Torre, S. Pareja López, S. Herrera, and C. A. Candela Uribe, “Comparative Study of AI Code Generation Tools: Quality Assessment and Performance Analysis,” LatIA, vol. 2, p. 104, Jul. 2024, doi: 10.62486/latia2024104.
P. Tembhekar, M. Devan, and J. Jeyaraman, “Role of GenAI in Automated Code Generation within DevOps Practices: Explore how Generative AI,” J. Knowl. Learn. Sci. Technol. ISSN 2959-6386 Online, vol. 2, no. 2, pp. 500–512, Oct. 2023, doi: 10.60087/jklst.vol2.n2.p512.
Mihir Mehta, “A comparative study of AI code bots: Efficiency, features, and use cases,” Int. J. Sci. Res. Arch., vol. 13, no. 1, pp. 595–602, Sep. 2024, doi: 10.30574/ijsra.2024.13.1.1718.
P. M. R. https://www.polarismarketresearch.com, “Generative AI Coding Assistants Market Trends Analysis Report, 2032.” Oct. 2024. Accessed: Dec. 06, 2024. [PDF]. Available: https://www.polarismarketresearch.com/industry-analysis/generative-ai-coding-assistants-market
Z. Chang and H. Sun, "Artificial intelligence-aided electrochemical sensors for capturing and analyzing fingerprint profiles of medicinal materials," International Journal of Electrochemical Science, vol. 19, no. 100887, 2024, doi: 10.1016/j.ijoes.2024.100887.
S. Rokhva, B. Teimourpour, and E. H. Soltani, "Computer vision in the food industry: Accurate, real-time, and automatic food recognition with pretrained MobileNetV2," Food and Human Behavior, vol. 19, no. 100378, 2024, doi: 10.1016/j.foohum.2024.100378.
N. S. Abdul et al., "Applications of artificial intelligence in the field of oral and maxillofacial pathology: A systematic review and meta-analysis," BMC Oral Health, vol. 24, no. 122, 2024, doi: 10.1186/s12903-023-03533-7.
K. L. Beck et al., "Development and evaluation of statistical and artificial intelligence approaches with microbial shotgun metagenomics data as an untargeted screening tool for use in food production," mSystems, vol. 9, no. 11, pp. 1–20, 2024, doi: 10.1128/msystems.00840-24.
P. Kumar and A. Maharajan, "Maritime Cybersecurity Leveraging Artificial Intelligence Mechanisms: Unveiling Recent Innovations and Projecting Future Trends," KSII Transactions on Internet and Information Systems, vol. 18, no. 10, pp. 3010–3039, 2024, doi: 10.3837/tiis.2024.10.010.
B. Yu, B. Wang, and Y. Zhang, "Application of artificial intelligence in coal mine ultra-deep roadway engineering—A review," Artificial Intelligence Review, vol. 57, no. 262, 2024, doi: 10.1007/s10462-024-10898-w.
Y. Wei, H. Du, Q. Hu, and H. Wang, "Optimizing Ship Draft Observation with Wave Energy Attenuation and PaddlePaddle-OCR in an Anti-Fluctuation Device," Journal of Marine Science and Engineering, vol. 12, no. 1865, 2024, doi: 10.3390/jmse12101865.
F. A. Panaite, M. Leba, and A. C. Ionica, "Assessing CNN Architectures for Estimating Correct Posture in Cruise Machinists," Eng, vol. 5, pp. 1785–1803, 2024, doi: 10.3390/eng5030094.
J.-M. Retrouvey and R. S. Conley, “Decoding Deep Learning applications for diagnosis and treatment planning,” Dent. Press J. Orthod., vol. 27, no. 5, p. e22spe5, 2022, doi: 10.1590/2177-6709.27.5.e22spe5.
L. Gonsioroski et al., “Artificial Intelligence Enabled Radio Propagation: Path Loss Improvement and Channel Characterization in Vegetated Environments,” J. Microw. Optoelectron. Electromagn. Appl., vol. 23, no. 1, p. e2024277600, 2024, doi: 10.1590/2179-10742024v23i1277600.
M. Arif, I. Ameer, N. Bölücü, G. Sidorov, A. Gelbukh, and V. Elangovan, “Mental Illness Classification on Social Media Texts Using Deep Learning and Transfer Learning,” Comput. Sist., vol. 28, no. 2, Jun. 2024, doi: 10.13053/cys-28-2-4873.
M. Stambuk-Castellano, I. Contreras-McKay, A. Neyem, O. Inzunza, N. E. Ottone, and M. Del Sol, “Plataforma de Software Educativa Gamificada: Experiencia con Estudiantes de Anatomía de la Universidad de La Frontera,” Int. J. Morphol., vol. 40, no. 2, pp. 297–303, 2022, doi: 10.4067/S0717-95022022000200297.
M. I. Jordan and T. M. Mitchell, “Machine learning: Trends, perspectives, and prospects,” Science, vol. 349, no. 6245, pp. 255–260, 2015, doi: 10.1126/science.aaa8415.
J. Lee, B. Bagheri, and H. A. Kao, “A cyber-physical systems architecture for industry 4.0-based manufacturing systems,” Manufacturing Letters, vol. 3, pp. 18–23, 2015, doi: 10.1016/j.mfglet.2014.12.001.
K. P. Murphy, Machine learning: A probabilistic perspective. MIT Press, 2012, doi: 10.5555/2380985.
K. T. Butler, D. W. Davies, H. Cartwright, O. Isayev, and A. Walsh, “Machine learning for molecular and materials science,” Nature, vol. 559, no. 7715, pp. 547–555, 2018, doi: 10.1038/s41586-018-0337-2.
D. Lazer et al., “Computational social science,” Science, vol. 323, no. 5915, pp. 721–723, 2009, doi: 10.1126/science.1167742.
C. J. Shallue and A. Vanderburg, “Identifying exoplanets with deep learning: A five-planet resonant chain around Kepler-80 and an eighth planet around Kepler-90,” The Astronomical Journal, vol. 155, no. 2, p. 94, 2018, doi: 10.3847/1538-3881/aa9e09.
V. Venkatasubramanian, “The promise of artificial intelligence in chemical engineering: Is it here, finally?,” AIChE Journal, vol. 65, no. 2, pp. 466–478, 2019, doi: 10.1002/aic.16489.
M. Reichstein et al., “Deep learning and process understanding for data-driven Earth system science,” Nature, vol. 566, no. 7743, pp. 195–204, 2019, doi: 10.1038/s41586-019-0912-1.
T. H. Davenport and R. Ronanki, “Artificial intelligence for the real world,” Harvard Business Review, vol. 96, no. 1, pp. 108–116, 2018, doi: 10.1109/EMR.2018.2861386.
J. Wang, H. Zhong, Z. Ma, Q. Xia, and C. Kang, “Review and prospect of integrated demand response in the multi-energy system,” Applied Energy, vol. 242, pp. 773–782, 2019, doi: 10.1016/j.apenergy.2019.03.174.
GitHub, "2023 State of Global Software Development," GitHub Report, 2023. [Online]. Available: http://www.comm.edu.faculty/sussman/sussmanpage.htm
McKinsey & Company, "The State of AI in 2023," McKinsey Report, 2023. [Online]. Available: http://www.comm.edu.faculty/sussman/sussmanpage.htm
Stack Overflow, "Developer Survey Results," Stack Overflow Insights, 2023. [Online]. Available: http://www.comm.edu.faculty/sussman/sussmanpage.htm
Forrester, "AI in Software Development," Forrester Research, 2023. [Online]. Available: http://www.comm.edu.faculty/sussman/sussmanpage.htm
Gartner, "AI Adoption in Organizations," Gartner Insights, 2023. [Online]. Available: http://www.comm.edu.faculty/sussman/sussmanpage.htm
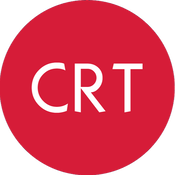
- Conceptualización
- Investigación
- Metodología
- Software
- Validación
- Redacción - borrador original
- Escritura, revisión y edición
- Conceptualización
- Curación de datos
- Análisis formal
- Adquisición de fondos
- Investigación
- Metodología
- Administración de proyecto
- Recursos
- Supervisión
- Visualización
- Escritura, revisión y edición
- Conceptualización
- Análisis formal
- Investigación
- Metodología
- Supervisión
- Validación
- Redacción - borrador original
Derechos de autor 2025 Innovación y Software

Esta obra está bajo licencia internacional Creative Commons Reconocimiento 4.0.
Los autores ceden en exclusiva el derecho de publicación de su artículo a la Revista Innovación y Software, que podrá editar o modificar formalmente el texto aprobado para cumplir con las normas editoriales propias y con los estándares gramaticales universales, antes de su publicación; asimismo, nuestra revista podrá traducir los manuscritos aprobados a cuantos idiomas considere necesario y difundirlos en varios países, dándole siempre el reconocimiento público al autor o autores de la investigación.